图书介绍
医学生物识别 数字化中医数据分析 英文版PDF|Epub|txt|kindle电子书版本网盘下载
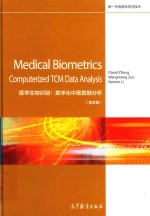
- David Zhang,Wangmeng Zuo,Naimin Li著 著
- 出版社: 北京:高等教育出版社
- ISBN:9787040428834
- 出版时间:2015
- 标注页数:398页
- 文件大小:48MB
- 文件页数:407页
- 主题词:数字技术-应用-中医诊断学-研究-英文
PDF下载
下载说明
医学生物识别 数字化中医数据分析 英文版PDF格式电子书版下载
下载的文件为RAR压缩包。需要使用解压软件进行解压得到PDF格式图书。建议使用BT下载工具Free Download Manager进行下载,简称FDM(免费,没有广告,支持多平台)。本站资源全部打包为BT种子。所以需要使用专业的BT下载软件进行下载。如BitComet qBittorrent uTorrent等BT下载工具。迅雷目前由于本站不是热门资源。不推荐使用!后期资源热门了。安装了迅雷也可以迅雷进行下载!
(文件页数 要大于 标注页数,上中下等多册电子书除外)
注意:本站所有压缩包均有解压码: 点击下载压缩包解压工具
图书目录
PART Ⅰ:DIAGNOSIS METHODS IN TRADITIONAL CHINESE MEDICINE3
Chapter 1 Introduction3
1.1 Diagnosis Methods in Traditional Chinese Medicine3
1.1.1 Tongue Diagnosis3
1.1.2 Pulse Diagnosis5
1.1.3 Breath Odor Diagnosis6
1.2 Computerized TCM Diagnosis7
1.2.1 Computerized Tongue Diagnosis7
1.2.2 Computerized Pulse Diagnosis11
1.2.3 Computerized Breath Odor Diagnosis14
1.3 Summary17
References17
PART Ⅱ:COMPUTERIZED TONGUE IMAGE ANALYSIS29
Chapter 2 Tongue Image Acquisition and Preprocessing29
2.1 Tongue Image Acquisition29
2.1.1 Requirement Analysis31
2.1.2 System Design and Implementation33
2.1.3 Performance Analysis43
2.2 Color Correction49
2.2.1 Color Correction Algorithms51
2.2.2 Evaluation of Correction Algorithms53
2.2.3 Discussion61
2.3 Summary67
References68
Chapter 3 Automated Tongue Segmentation73
3.1 Bi-Elliptical Deformable Contour73
3.1.1 Bi-Elliptical Deformable Template for the Tongue74
3.1.2 Combined Model for Tongue Segmentation78
3.1.3 Results and Analysis84
3.2 Snake with Polar Edge Detector91
3.2.1 The Segmentation Algorithm91
3.2.2 Experimental Results99
3.3 Gabor Magnitude-based Edge Detection and Fast Marching104
3.3.1 2D Gabor Magnitude-based Edge Detection105
3.3.2 Contour Detection Using Fast Marching and Active Contour Model109
3.3.3 Experimental Results111
3.4 Summary114
References114
Chapter 4 Tongue Image Feature Analysis117
4.1 Color Feature Analysis117
4.1.1 Exploratory Tongue Color Analysis118
4.1.2 Statistical Analysis of Tongue Color Distribution124
4.2 Tongue Texture Analysis143
4.3 Tongue Shape Analysis144
4.3.1 Shape Correction144
4.3.2 Extraction of Shape Features149
4.3.3 Tongue Shape Classification153
4.4 Extraction of Other Local Pathological Features158
4.4.1 Petechia158
4.4.2 Tongue Crack160
4.4.3 Tongueprint160
4.4.4 Sublingual Veins161
4.5 Summary162
References163
Chapter 5 Computerized Tongue Diagnosis167
5.1 Bayesian Network for Computerized Tongue Diagnosis167
5.1.1 Quantitative Pathological Features Extraction167
5.1.2 Bayesian Networks169
5.1.3 Experimental Results171
5.2 Diagnosis Based on Hyperspectral Tongue Images178
5.2.1 Hyperspectral Tongue Images179
5.2.2 The SVM Classifier Applied to Hyperspectral Tongue Images180
5.2.3 Experimental Results183
5.3 Summary186
References187
PART Ⅲ:COMPUTERIZED PULSE SIGNAL ANALYSIS191
Chapter 6 Pulse Signal Acquisition and Preprocessing191
6.1 Pressure Pulse Signal Acquisition191
6.1.1 Application Scenario and Requirement Analysis192
6.1.2 System Architecture193
6.1.3 Multi-Channel Pulse Signals201
6.2 Baseline Wander Correction of Pulse Signals206
6.2.1 Detecting the Onsets of Pulse Wave207
6.2.2 Wavelet Based Cascaded Adaptive Filter209
6.2.3 Results on Actual Pulse Signals221
6.3 Summary223
References224
Chapter 7 Feature Extraction of Pulse Signals227
7.1 Spatial Feature Extraction227
7.1.1 Fiducial Point-based Methods227
7.1.2 Approximate Entropy229
7.2 Frequency Feature Extraction230
7.2.1 Hilbert-Huang Transform230
7.2.2 Wavelet and Wavelet Packet Transform232
7.3 AR Model234
7.4 Gaussian Mixture Model236
7.4.1 Two-term Gaussian Model236
7.4.2 Feature Selection240
7.4.3 FCM Clustering242
7.5 Summary242
References243
Chapter 8 Classification of Pulse Signals245
8.1 Pulse Waveform Classification245
8.1.1 Modules of Pulse Waveform Classification246
8.1.2 The EDFC and GEKC Classifiers251
8.1.3 Experimental Results255
8.2 Arrhythmic Pulses Derection257
8.2.1 Clinical Value of Pulse Rhythm Analysis257
8.2.2 Automatic Recognition of Pulse Rhythms259
8.2.3 Experimental Results272
8.3 Combination of Heterogeneous Features for Pulse Diagnosis274
8.3.1 Multiple Kernel Learning275
8.3.2 Experimental Results and Discussion279
8.4 Summary282
References283
PART Ⅳ:COMPUTERIZED ODOR SIGNAL ANALYSIS289
Chapter 9 Breath Analysis System:Design and Optimization289
9.1 Breath Analysis289
9.2 Design of Breath Analysis System291
9.2.1 Description of the System291
9.2.2 Signal Sampling and Preprocessing296
9.3 Sensor Selection299
9.3.1 Linear Discriminant Analysis299
9.3.2 Sensor Selection in Breath Analysis System304
9.3.3 Comparison Experiment and Performance Analysis314
9.4 Summary317
References317
Chapter 10 Feature Extraction and Classification of Breath Odor Signals321
10.1 Feature Extraction of Odor Signals321
10.1.1 Geometry Features322
10.1.2 Principal Component Analysis324
10.1.3 Wavelet Packet Decomposition324
10.1.4 Gaussian Function Representation325
10.1.5 Gaussian Basis Representation331
10.1.6 Experimental Results334
10.2 Common Classifiers for Odor Signal Classification336
10.2.1 K Nearest Neighbor337
10.2.2 Artificial Neural Network337
10.2.3 Support Vector Machine337
10.3 Sparse Representation Classification338
10.3.1 Data Expression338
10.3.2 Test Sample Representation by Training Samples339
10.3.3 Samples Sampling Errors340
10.3.4 Voting Rules341
10.3.5 Identification Steps342
10.4 Support Vector Ordinal Regression342
10.4.1 Problem Analysis342
10.4.2 Basic Idea of Support Vector Regression343
10.4.3 Support Vector Ordinal Regression344
10.4.4 The Dual Problem345
10.4.5 Identification Steps346
10.5 Evaluation on Classification methods347
10.5.1 Evaluation on SRC347
10.5.2 Evaluation on SRC351
10.6 Summary355
References355
Index359